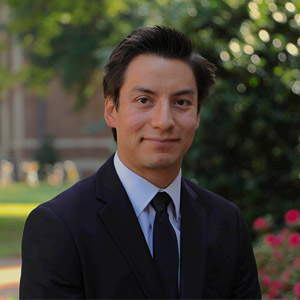
Job market paper
A Wild Bootstrapped t-test Robust to all Identification Categories
In this paper, we introduce an asymptotically valid wild bootstrapped t-test, which provides robust inference for models with unknown identification category. Under weak identification, the wild bootstrap needs to be constructed using residuals imposing lack of identification, because the usual regression residuals are non-consistent. The robust t-test has better small sample properties compared to the asymptotic approximation counterpart and is simpler to estimate in practice, especially when the underlying Gaussian process has an unknown form and/or is high dimensional. A simulation exercise shows the benefits of using a robust t-test exposing the large-size distortions of the standard t-test when weak identification is present. In our empirical application, we consider the mixed data sampling (MIDAS) model proposed by Ghysels et al. (2005) to study the risk-return trade-off. Contrary to their results, the robust t-test indicates that the estimators of the MIDAS model are not significant, which suggests that the proposed functional form has low explanatory and predictive power in the study of the risk-return trade-off.
Fields
18, 21, 21, 0, Financial Econometrics, High Frequency Econometrics, Time-Series Econometrics, Asset PricingContact information
- jacamp22@live.unc.edu
- (919) 904-5783
- Website
- CV
- Gardner Hall CB 3305
- University of North Carolina
- Chapel Hill, NC 27599-3305
Letter writers
- Jonathan B. Hill
- Peter R. Hansen
- Ju Hyun Kim
- Helen V. Tauchen